Technical Articles, Integration
DeepSeek: an opportunity for Efficient AI
Feb 18, 2025
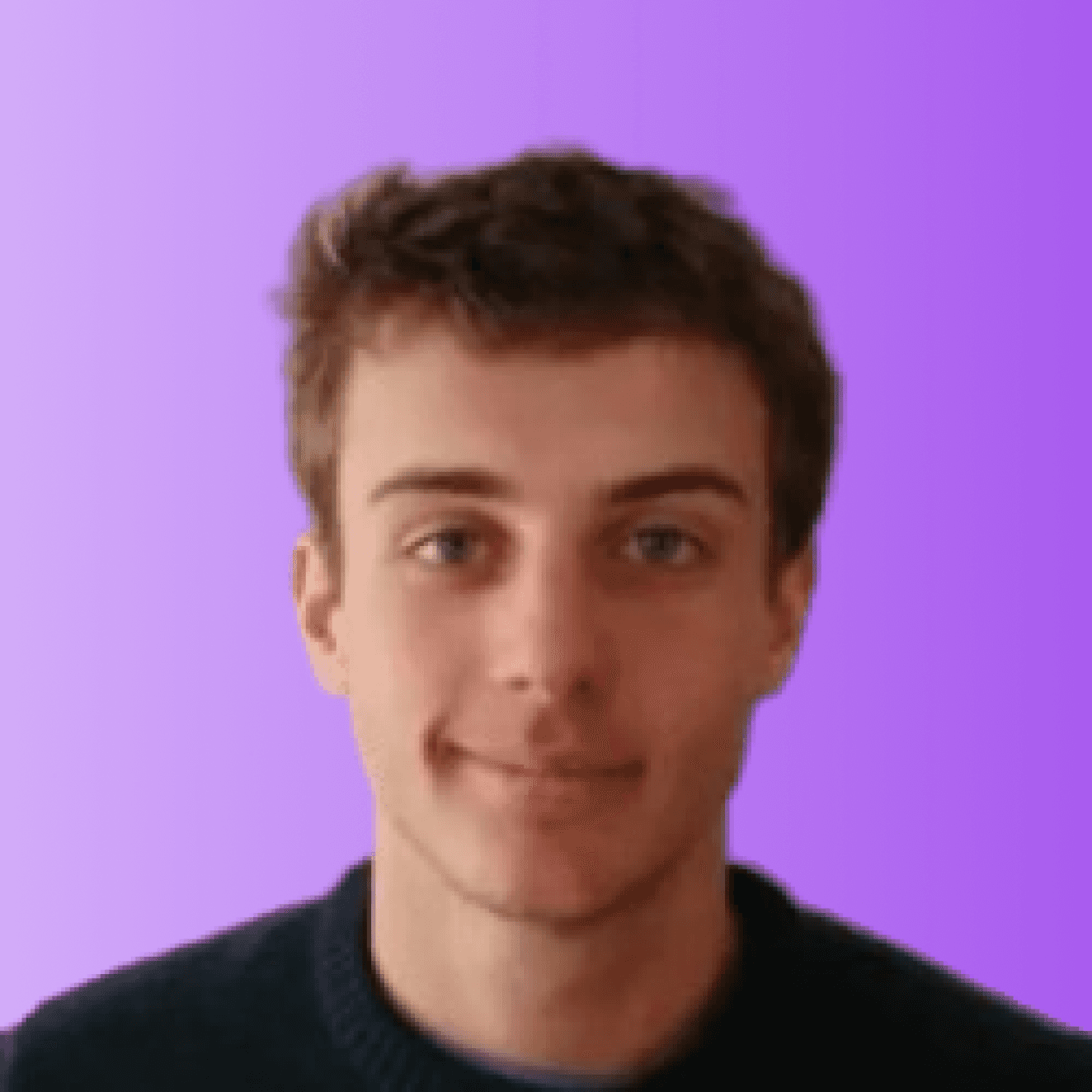
Bertrand Charpentier
Cofounder, President & Chief Scientist

Quentin Sinig
Go-to-Market Lead
DeepSeek’s Breakthrough: Hype vs. Reality
In recent news, DeepSeek has sparked huge interest in the AI community. Beyond the hype and all the noise, we wanted to take a moment to digest the news, see how it impacted our discussions with customers, and evaluate its impact on AI efficiency.
Well, TL;DR: it’s an opportunity. People are starting to realize that we can build great AI in a more frugal fashion and that this is accessible to organizations of all sizes. This shift is exactly what we’ve been advocating at Pruna AI, so we welcome DeepSeek’s momentum as a positive development. Yet, inference is still costly, and the reasoning model consumes almost 2× more energy than Llama 3.3.
This blog aims to explain the context around DeepSeek’s latest progress and clarify key misconceptions and takeaways.
Wait, What’s DeepSeek Again?
DeepSeek is an AI company founded in 2023. It has around ~200 employees, most of them high-tech profiles contributing to tech reports. The company actively works on releasing multiple AI models, mostly large language models specialized in coding, math, or generalist models. These models are mostly based on existing Machine Learning techniques (e.g., variants of Llama architecture, Proximal Policy Optimization, Mixture of Experts, Chain of Thought). While these techniques do not demonstrate significant scientific novelty, DeepSeek recently raised interest in smartly combining them with engineering efforts to achieve never-achieved training efficiency.
A major example is the DeepSeek R1 model, which was estimated to cost $5.5M for training compared to $100M for GPT-4, showing many that training LLMs was more affordable than the general public thought. Following this news, the market value of many hardware and energy companies crashed by multiple hundred billion. It also sparked reactions from AI experts like Boris Gamazayvhikov, Gary Marcus, Thomas Wolf, Dario Amodei, Sasha Lucioni, and many more… each bringing reasonable perspectives.
What Did We Really Learn from DeepSeek?
Open-source drives AI adoption.
Deploying AI models in production is complicated since it requires full trust in their behavior, while these models remain black boxes that can demonstrate abnormal behavior, such as adversarial attacks (see. this blog). While this does not fully solve the trust problem, one way to facilitate AI adoption is to share as many technical details as possible, like papers, tech reports, and model weights, while still protecting critical proprietary elements. In that spirit, DeepSeek shared a technical report and many model variations for the community to build on. Note that this technique is not new. Llama and Mistral already followed a similar path in the past, leading to a wave of adoption in the AI community.
AI development doesn’t rely on GAFAM alone.
DeepSeek’s progress mostly shows that both GAFAM and emerging AI companies (like Hugging Face, Mistral… and Pruna AI 😉), have the talent to efficiently leverage the huge body of shared research and knowledge in AI. More specifically, this talent is not limited to the US. DeepSeek is another proof that competitive AI development is also happening in Europe and Asia, where teams can develop strong offerings. For example, DeepSeek pricing is below $2 for 1M tokens (pricing here) compared to more than $15 for GPT (pricing here).
However, it is important to keep in mind that Big Tech still has huge advantages in terms of data and infrastructure. These companies can scale training and deploy high-quality AI models to massive audiences. That’s why Mistral AI is investing billions in building data centers in France to compete at scale (source). It’s worth mentioning that the DeepSeek models do not break the so-called scaling laws. In other words, companies that can afford more compute, data, and parameters can still push performance further. One key takeaway is that it remains difficult for companies without dedicated AI teams to keep up with the fast pace of AI development.
Compute cost is not the only variable.
Compute costs for AI training remain huge. While DeepSeek optimized the training cost of a single model instance down to $5.5M compared to $100M for GPT-4, it still remains out of reach for most companies looking to train their own models. Beyond training, there are other important cost factors:
Development costs, including the salaries of the 180+ top ML engineers who contributed to the tech report.
Inference costs to serve millions of users, with at least 10 H100 GPUs required just to fit a single copy of the 720GB DeepSeek-R1 model at once.
Energy consumption, since DeepSeek-R1, as a reasoning model using Chain of Thought, requires 87% more energy than Llama 3.3.
Just like we succeeded in reducing DBRX's minimum requirements from 4 GPUs to just 1 A100 GPU, making it significantly cheaper and more scalable for users, we aim to deliver even more efficient DeepSeek models to the community (source).
Efficient hardware is just one angle of “Efficient AI”.
The market crash of hardware and energy companies following DeepSeek’s announcement made one thing clear. Simply scaling up hardware and compute power is not enough for widespread AI adoption. DeepSeek's technical report highlights a point that many in the AI community already suspected. The right combination of models, training methods, and inference strategies is just as important as raw compute power. This aligns with past research, which has shown that algorithmic innovations often drive the biggest efficiency gains.
AI’s biggest bottleneck? Knowledge, not compute.
While many of the techniques used in DeepSeek-R1, like Mixture of Experts, GRPO, and Distillation, are not new, it was unclear to many that this level of efficiency was even possible.
Even for experts, achieving a high level of efficiency in training large language models is complicated. DeepSeek’s real achievement lies in finding the right combination of data collection and algorithmic methods. The solution itself may seem simple, but the real challenge is understanding, applying, and optimizing these techniques effectively.
Even with access to technical reports, many companies still face the barrier of acquiring the specialized knowledge required to replicate state-of-the-art AI results with smaller teams. This confirms that even in early 2025, the "lack of AI skills, expertise, or knowledge" remains one of the top three reasons slowing down AI adoption, as highlighted in the IBM Global AI Adoption Index. It is a trend we spotted as early as 2022, and it still holds true today.
Final Takeaways
While the DeepSeek publication led to multiple wrong conclusions among non-expert audiences, expert reactions align on key takeaways.
Open-source collaboration accelerates AI adoption. Transparency, research papers, and shared model weights have proven to drive real-world usage, as seen with DeepSeek, Llama, and Mistral.
Smaller AI players can compete, but Big Tech still has the advantage of scale. While companies like DeepSeek, Mistral, and Pruna have shown that efficiency-driven engineering can unlock significant progress, Big Tech still benefits from superior data and infrastructure.
AI remains expensive beyond just compute costs. Training and deployment require not only computational resources, but also energy efficiency and scalable inference to remain viable.
Hardware alone is not the key to AI efficiency. Algorithmic innovations play an equally critical role, and DeepSeek’s progress highlights that smart engineering choices can drive major improvements.
The AI knowledge gap remains a top barrier. Even in early 2025, the "lack of AI skills, expertise, or knowledge" continues to slow down adoption (IBM Global AI Adoption Index).
At Pruna AI, we focus on these exact challenges to make AI more efficient, accessible, and sustainable. That is why we see DeepSeek’s momentum as a positive step forward 🙂
References
DeepSeek-R1: Incentivizing Reasoning Capability in LLMs via Reinforcement Learning
Pour DeepSeek: Démystifier les cinq principaux malentendus et interpréter la vérité.
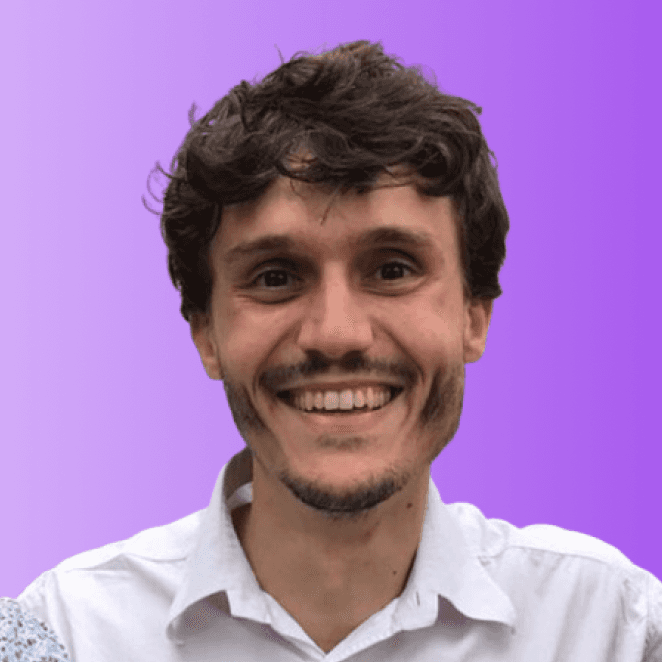
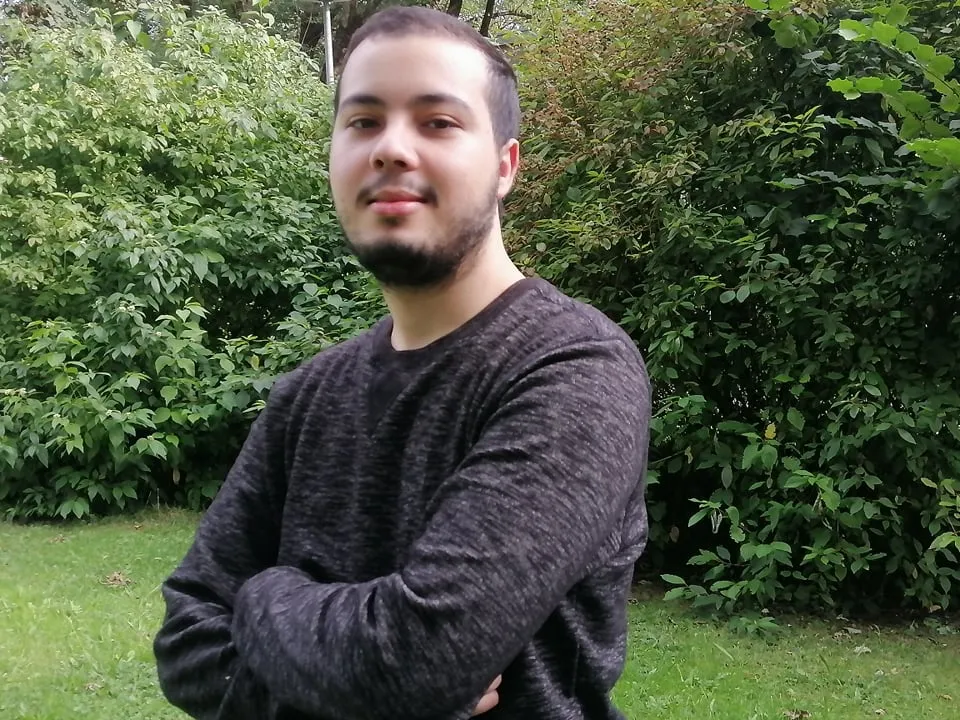
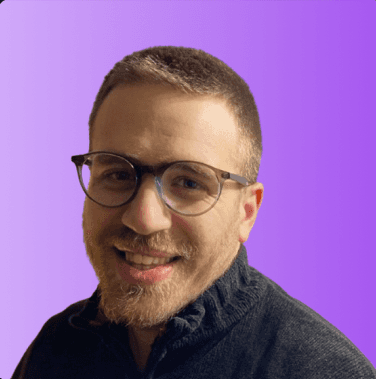
・
Feb 18, 2025
Subscribe to Pruna's Newsletter